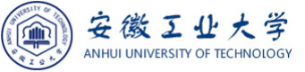
报告题目:Imbalanced Data Learning: Always Needed?
报告人:张晓明 教授
报告时间:2022年12月21日下午14:00-15:00 (中国Beijing GMT +8.00)
报告地点:(线上)#腾讯会议:596-726-773
报告对象:感兴趣的教师、研究生等
主办单位:英国ladbrokes官方网站
报告人简介:
张晓明(CHEUNG, Yiu-ming)香港浸会大学(浸大)人工智能讲席教授、研究生院副院长及浸大深圳研究院院长,IEEE Fellow, AAAS Fellow, IET Fellow以及英国计算机学会Fellow,列入2020, 2021及2022年史丹福大学所发表的人工智能与图像处理专业领域世界顶尖科学家排名前1%。IEEE计算智能学会香港分会始创者及前任主席, IEEE计算机学会智能信息学委员会(TCII)现任主席,香港浸会大学计算和理论科学研究所副所长。长期从事人工智能、模式识别、图像及视频处理、函数优化等领域研究,在相关国际著名期刊及学术会议上,如 IEEE Transactions on Pattern Analysis and Machine Intelligence, IEEE Transactions on Information Forensics and Security, CVPR, IJCAI, AAAI等已发表论文逾250余篇。2011年及2021年两次获得香港浸会大学计算机科学系最佳研究奖,2020年获选IEEE 计算智能学会Distinguished Lecturer。主持包括香港研究资助局、国家自然科学基金以及联合基金等三十余项。获2017年第七届香港创新科技成就大奖香港创新发明奖金牌,2018年再次荣获第46届日内瓦国际发明展评判嘉许特别金奖以及罗马尼亚优异奖。曾担任包括IJCAI, ACML, ICIP, ICPR, ICDM, WI 在内的多个国际著名会议的程序委员会主席,组织委员会主席,领域主席等。担任IEEE Transactions on Emerging Topics in Computational Intelligence期刊的主编(2023-),同时也担任若干一流国际期刊的副主编。
Abstract:
In many practical problems, the number of data forming difference classes can be quite imbalanced, which could make the performance of the most machine learning methods become deteriorate to a certain degree. In general, the problem of learning from imbalanced data is nontrivial and challenging in the fields of machine learning and data science, which has attracted growing attentions in recent years. In this talk, we will first introduce the class imbalanced learning and its related techniques. Then, whereas the class imbalance is quite common from the practical perspective, we will attempt to address the problem naturally arisen: When should such a class imbalance be taken into account in the machine learning tasks? Furthermore, considering the unavailability of class labels in some applications, this talk will also present our recent work on imbalanced data clustering in an unsupervised learning environment. Lastly, some challenges in this field will be introduced.
欢迎全校师生踊跃参加!